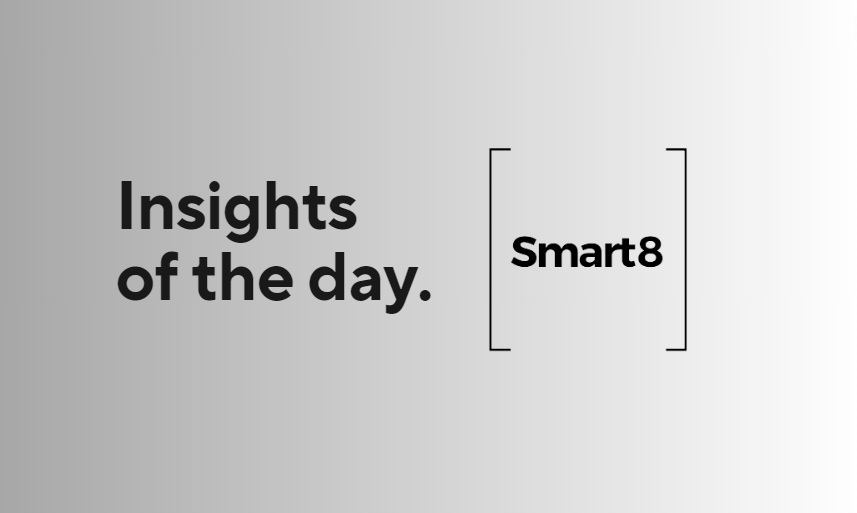
Gain valuable insights and practical knowledge that will set you apart from the competition. Whether you're just starting out or looking to level up your existing skillset, our comprehensive guide has got you covered.
Data-driven decisions are business decisions that are based on the analysis and interpretation of data. This approach to decision-making relies on quantitative and qualitative data to guide the decision-making process, rather than relying solely on intuition or personal experience. By using data to inform decisions, businesses can make more informed, strategic choices that are likely to lead to better outcomes.
Data-driven decisions are made possible through the use of data analytics tools and techniques, which enable businesses to collect, organize, and analyze large amounts of data. This data can come from a variety of sources, including customer feedback, sales data, market trends, and more. By analyzing this data, businesses can identify patterns and trends that can inform their decisions, such as which products or services are most popular, which marketing strategies are most effective, and which areas of the business may need improvement.
The benefits of data-driven decisions include increased accuracy, efficiency, and productivity. By relying on data rather than intuition, businesses can make decisions that are less prone to error and more likely to be successful. Additionally, data-driven decisions can help businesses to identify areas for improvement and optimize their operations, leading to increased efficiency and productivity. Overall, data-driven decisions can help businesses to gain a competitive advantage and achieve their goals more effectively.
Data-driven decision making is a process of making business decisions based on data analysis. It involves using data to identify patterns, trends, and relationships that can inform business decisions.
The first step in data-driven decision making is to identify a problem or opportunity that needs to be addressed. This involves defining the problem or opportunity and determining what data is needed to address it.
Once the problem or opportunity has been identified, the next step is to collect and analyze data related to it. This may involve collecting data from various sources, such as customer feedback, sales data, or market trends.
After collecting and analyzing data, the next step is to interpret and visualize it. This involves using data visualization tools and techniques to present the data in a way that is easy to understand and interpret.
With the data interpreted and visualized, the next step is to make a decision based on the data. This involves using the data to identify patterns, trends, and relationships that can inform the decision.
Once a decision has been made, it's important to implement it and monitor its effectiveness. This involves tracking key performance indicators (KPIs) and adjusting the decision as needed based on new data.
Let's take the example of a retail company that wants to optimize its inventory management. The company has been relying on intuition and historical data to manage its inventory, but it wants to improve its accuracy and reduce waste. Here's how it can use data-driven decision making to achieve this goal:
The company starts by collecting data on customer demand, sales patterns, and inventory levels. It uses data analytics tools to analyze this data and identify patterns and trends. For example, it finds that customer demand for a particular product is highest during the winter months, and that inventory levels for this product are typically low during this time.
Based on the data analysis, the company identifies a problem: it is consistently running out of stock for this popular product during the winter months, leading to lost sales and customer dissatisfaction. It also identifies an opportunity: by optimizing its inventory management, it can reduce waste and improve profitability.
The company uses the insights from the data analysis to make informed decisions about inventory management. It decides to increase its inventory levels for the popular product during the winter months, and to implement a more efficient inventory management system to reduce waste. It also decides to offer promotions and discounts during this time to maximize sales.
The company implements the new inventory management system and monitors its effectiveness using data analytics. It tracks key performance indicators (KPIs) such as inventory levels, sales, and customer satisfaction. Based on the data, it makes adjustments to its inventory management strategy as needed.
The company sees a significant improvement in its inventory management and sales. It reduces waste and increases profitability, while also improving customer satisfaction. The data-driven approach allows it to make informed decisions that drive growth and success.
In this case study, we saw how a retail company used data-driven decision making to optimize its inventory management and drive business success. By collecting and analyzing data, identifying patterns and trends, and making informed decisions, the company was able to improve its profitability and customer satisfaction. This approach can be applied to any business looking to make informed decisions that drive growth and success.